Deep Learning
Deep Learning
Deep Learning is a type of machine learning method based on artificial neural networks. The algorithms used in Deep Learning are usually very complex and consist of several layers of neural networks.
Neural networks consist of a large number of neurons that are connected to each other and pass data from one layer to the next.
An artificial neural network learns by being trained on a large amount of sample data. During the training process, the weights and connections between neurons are adjusted to better fit the network to the sample data. After training, the network can be applied to new, unknown data to perform predictions or classifications.
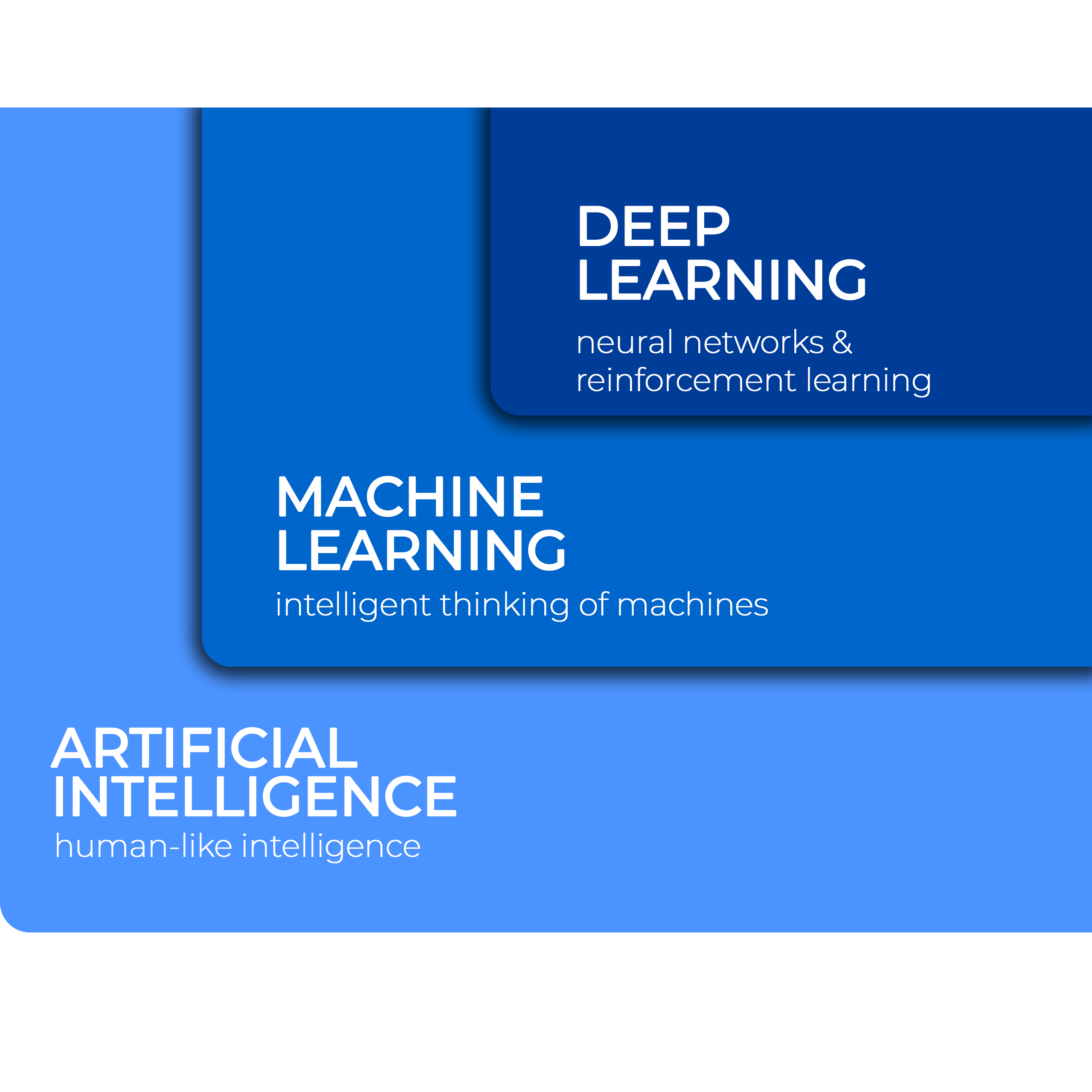
Deep Learning use at EYYES
We use Deep Learning to solve complex image processing problems in the railway, automotive and industrial sectors, where environmental influences and variations can never be fully controlled.
Image processing has achieved a real leap in quality and robustness through Deep Learning. As a result, object recognition can now be carried out in real time. Cameras offer clear advantages over other sensors such as radar, lidar or similar. Our object recognition and classification technology enables new use cases for driving assistance systems and autonomous driving.
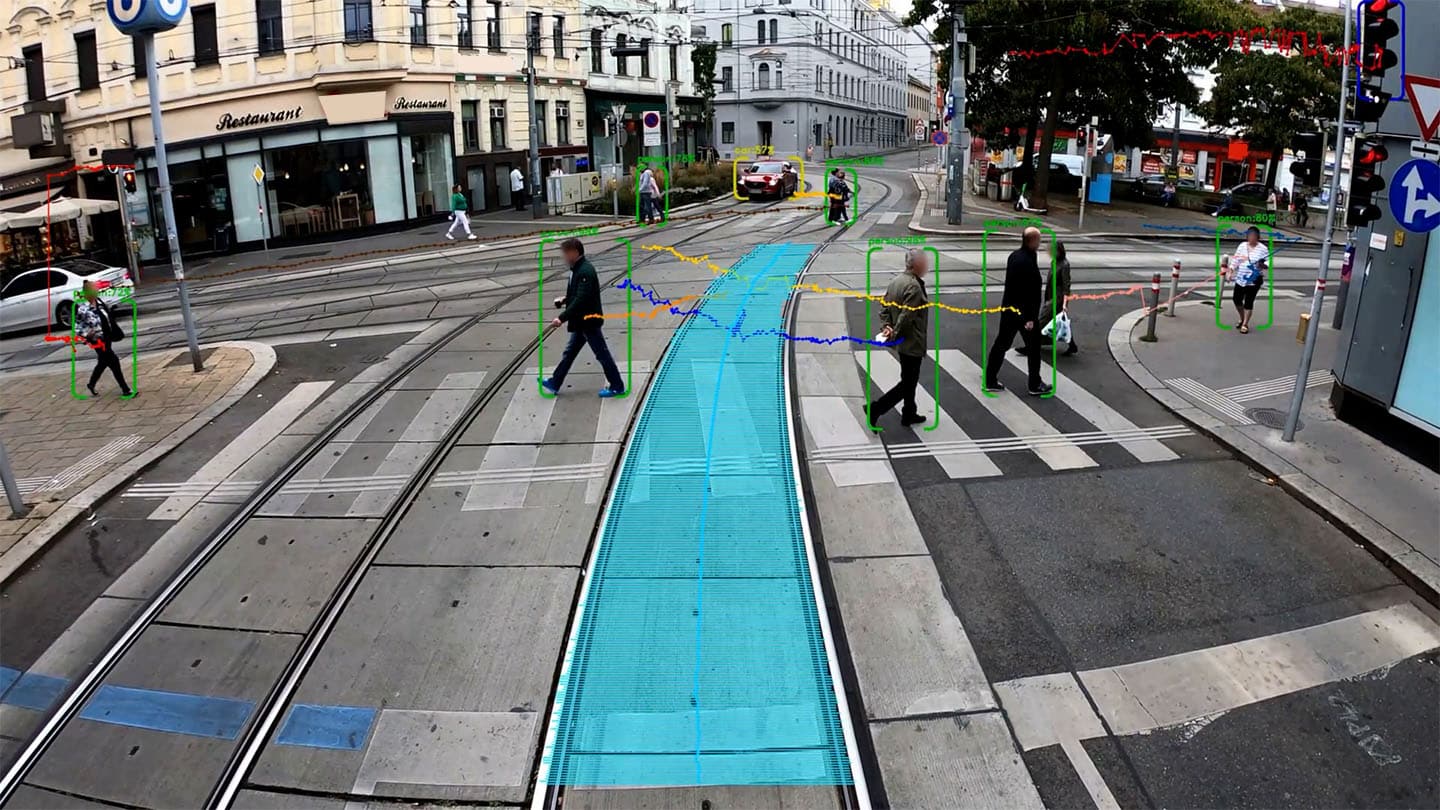
[servicebox_sc id=20351]
EYYESNet
The EYYESNet is a neural network for visual object recognition from images, covering the most important traffic-related classes (person, bicycle/motorcycle, car, truck/bus, train/tram) for automotive and railway solutions. It achieves the detection quality of a YOLO v3 object detector with a processing complexity of only 5%, enabling higher frame rates and multi-sensor setup with the same processing hardware.
EYYESNet can be used with traditional AI inference engines or the powerful EYYES Layer Processing Unit. It has been developed in a step-by-step training process over 5 years, acquiring more than 5 PB of real image data, plus more than 10 PB of synthetic data for different weather and environmental conditions.
Detection of:
- Pedestrians (adults, children, …)
- Cyclists
- Cars, trucks, trains
- Traffic signal detection
- Traffic sign recognition
- Lane detection
EYYESNet Use Cases
The EYYESNet can be used for the following applications:
Digital exterior rear view mirror with AI for proactive driver warnings in all traffic situations.
UNECE R151 BSIS: Blind Spot Information System with a minimum levels of faults positive alarms
UNECE WP29 MOIS: Move Of Information System
Bird’s eye view
Lane Change Assist
Detection of moving objects such as pedestrians, cyclists, cars, trucks in order to maintain distances and intervene in driver assistance in case of danger